Enhancing Predictive Maintenance at Nordic Sugar: Insights into Steam Dryer Optimization
Nordic Sugar, a key player in the sugar production industry and a part of the Nordzucker Group, has been pioneering innovative maintenance strategies at its Nakskov plant in Denmark. As part of their continuous improvement efforts, they have embarked on a significant project to implement predictive maintenance on their steam dryer, a crucial component in their sugar production process. This initiative, led by Anders Jørgensen-Juul, Head of Projects, is setting new benchmarks in the industry.
Nordzucker Group operates several factories across Europe and Australia, employing around 4.000 people. The Nakskov plant, where Mr. Jørgensen-Juul is based, has been at the forefront of integrating advanced technologies into its operations. “We are part of a much larger group, but each plant operates with a degree of autonomy that allows us to innovate and implement new technologies,” says Jørgensen-Juul.
The Role of the Steam Dryer
In the sugar production process, the steam dryer plays a vital role in drying beet pulp. Traditionally, high-temperature drying methods were used, which consumed a lot of energy and made it difficult to reuse the heat. Modern steam dryers, however, operate on a fluidized bed principle, using hot air heated by primary steam to dry the pulp. This method not only improves energy efficiency but also allows for the recovery and reuse of secondary steam, thereby enhancing sustainability.
“Maintaining the pressure inside the dryer is crucial,” explains Mr. Jørgensen-Juul. “We typically have about 3,5 to 4 bars inside, while the ambient pressure outside is much lower. Our challenge was with the outlet valve, which suffered from multiple breakdowns.”
Identifying the problem
The unpredictable failures of the outlet valve posed a significant operational risk. “We couldn’t predict when it would break down, and it often happened at inopportune times, leading to unscheduled downtimes,” says Jørgensen-Juul. “This unpredictability was costly and disruptive.”
To address this, Nordic Sugar decided to implement a predictive maintenance strategy using machine learning. “We realized there was a business case for investing in high-end predictive maintenance technologies, even though they are expensive,” notes Jørgensen-Juul.
Implementing Machine Learning for Predictive Maintenance
The implementation process began with extensive data collection. “We gathered data from various sensors and our Distributed Control System (DCS), combining it with historical failure data,” Jørgensen-Juul explains. “The hard part was transforming this data so it could be compiled and used to train the machine learning model.” He also emphasized the importance of data quality. “Good quality data is the foundation of any successful machine learning project. It’s not just about having a lot of data, but ensuring it’s accurate and relevant,” he notes.
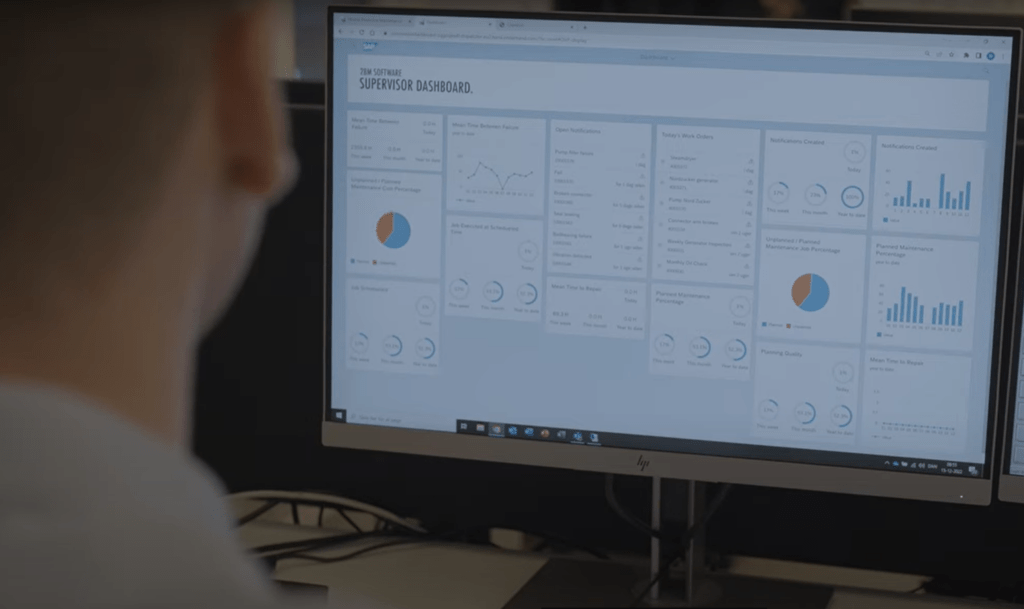
In a next phase, the model was trained to recognize patterns that indicated potential failures. “The multidimensional model looks at patterns across different variables and predicts when a breakdown might occur,” says Jørgensen-Juul. “This allows us to estimate the remaining useful life of the component and schedule maintenance accordingly.”
Results and Learnings
The results of this initiative have been promising. In the first year, the model’s predictions were off by only 13 days. “This was a significant improvement,” says Jørgensen-Juul. “It allowed us to make more informed decisions about when to perform maintenance.”
However, the project was not without its challenges. “In 2023, our predictions were off by 35 days, which was a setback. We realized that small modifications to the equipment by our technicians had affected the model’s accuracy. The ML algorithm was not trained to consider structural changes in the machine itself.. We now understand that even small changes can seriously disrupt the accuracy of the RUL prediction.” Despite this, the project has yielded valuable insights. “We’ve learned a lot about the sensitivity of machine learning models and the importance of maintaining a stable system,” says Jørgensen-Juul. “These learnings are invaluable as we continue to refine our approach.”
One of the primary motivations for this project was to create a strong business case for predictive maintenance. “Normally, we run a campaign of 110 days and only see a breakdown after 85 or 90 days,” explains Jørgensen-Juul. “In the past, we would replace the rotor and internal components at the time of the breakdown and again after the campaign ends, just to ensure we could complete the following campaign without issues. This was not cost-effective.”
By using predictive maintenance, Nordic Sugar aimed to extend the life of these components. “The goal was to avoid premature replacements and reduce the number of spare parts used. Our first-year success showed that we could predict breakdowns within a 13-day margin, which is a huge improvement,” notes Jørgensen-Juul. “Even with the setback in the second year, we gained critical insights into improving our models and processes.”
Future Applications and Sustainability
Looking ahead, Nordic Sugar plans to expand the use of predictive maintenance. “We’re cautious about where we apply machine learning due to its resource demands,” explains Jørgensen-Juul. “But for critical components with high failure costs and limited alternatives for condition-based maintenance, it’s worth the investment.”
The project also aligns with Nordic Sugar’s sustainability goals. ” Sustainability is really gained through predictive maintenance. It helps us extend the life of our equipment, reducing the need for spare parts and minimizing waste,” says Jørgensen-Juul. “It’s a key part of our strategy to improve resource efficiency and reduce our environmental impact. The longer we can wait until replacing parts, the more resources we conserve. Maintenance staff, even skilled, can only approximate so much, but machine learning can narrow down the accuracy to about two percent.”
Learning opportunity
The success at Nordic Sugar’s Nakskov plant offers valuable lessons for the broader industry. “Predictive maintenance can significantly enhance operational efficiency and sustainability,” says Jørgensen-Juul. “However, it’s essential to have a clear business case and a stable system. Also, it’s crucial to involve technical personnel in the process since they are the only ones who can link behaviour and failures of the equipment to the trends in the data.”
Anders Jørgensen-Juul will be presenting the Nordic Sugar case in detail at the Asset Performance 2024 conference in Antwerp, Belgium, on November 13th and 14th, 2024. His presentation will offer a deeper dive into the challenges, solutions, and learnings from the project, providing valuable insights for professionals in the maintenance and reliability fields. Don’t miss this opportunity to learn from a leading expert in the industry. Register now for Asset Performance 2024 and be part of the conversation shaping the future of maintenance and asset management.