Drive Smarter Maintenance and Better Operating Decisions
By collecting, interpreting and applying the right data.
With the rise of the Fourth Industrial Revolution (Industry 4.0), a new world of possibilities has opened for manufacturers.
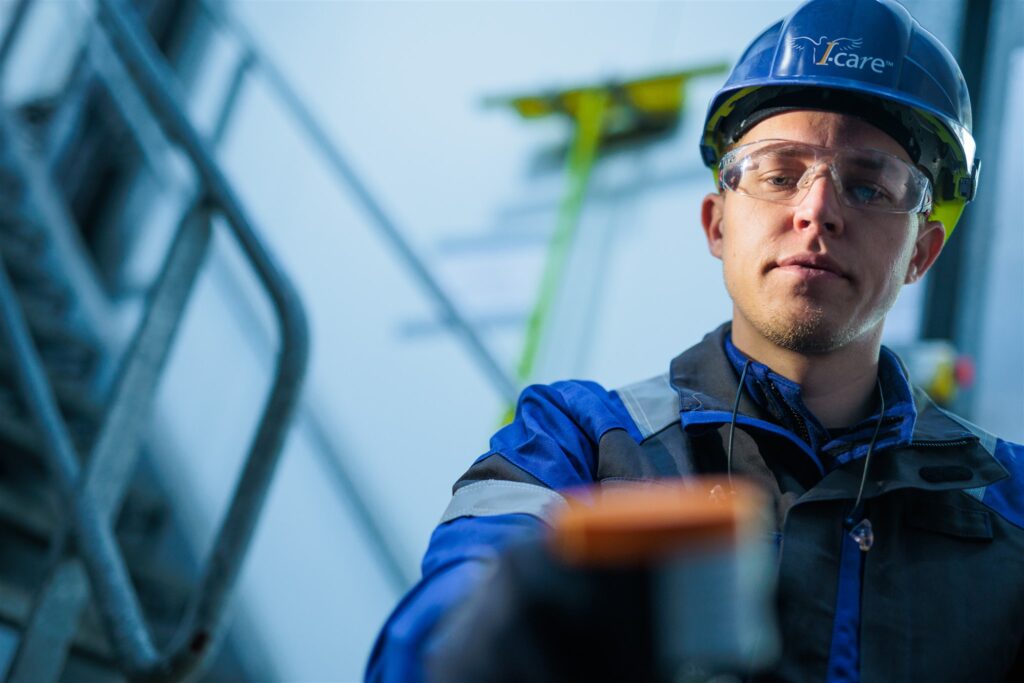
Given this extraordinary evolution, it’s inevitable that the shift must involve leveraging all the value inherent in available data. Furthermore, statistics support that outcome. In 2019, a joint study was conducted by Deloitte and the Manufacturer’s Alliance for Productivity and Innovation (MAPI) to make the business case for investing in smart factory initiatives to drive better outcomes.
Among the findings, the study found that firms that implement smart factory initiatives report an average of 10-12% gains in manufacturing output, factory utilization and labor productivity. Despite this seismic shift, many firms are still struggling with IT challenges, ranging from aging infrastructure to incomplete (or ineffective) utilization of the cloud. Additionally, cybersecurity remains a huge concern, with risks ranging from operational downtime and physical damage to theft of intellectual property and sensitive data. In 2016, IBM identified the manufacturing sector as being one of industries most heavily targeted by cyberattackers. As of February 2021, manufacturing operations were still on cyberattackers “most wanted list.”
Further compounding the problem, manufacturing leaders often don’t realize that the information to address all these challenges is already available to them. Trillions of data points are being generated every day by an array of industrial activities, facility, and machine activities. All of them can be collected, aggregated, analyzed, and interpreted to provide deeper, richer insights never before possible. Reaping full value from this data not only mitigates risk; it also boosts productivity and profit.
EXTRACTING THE VALUE
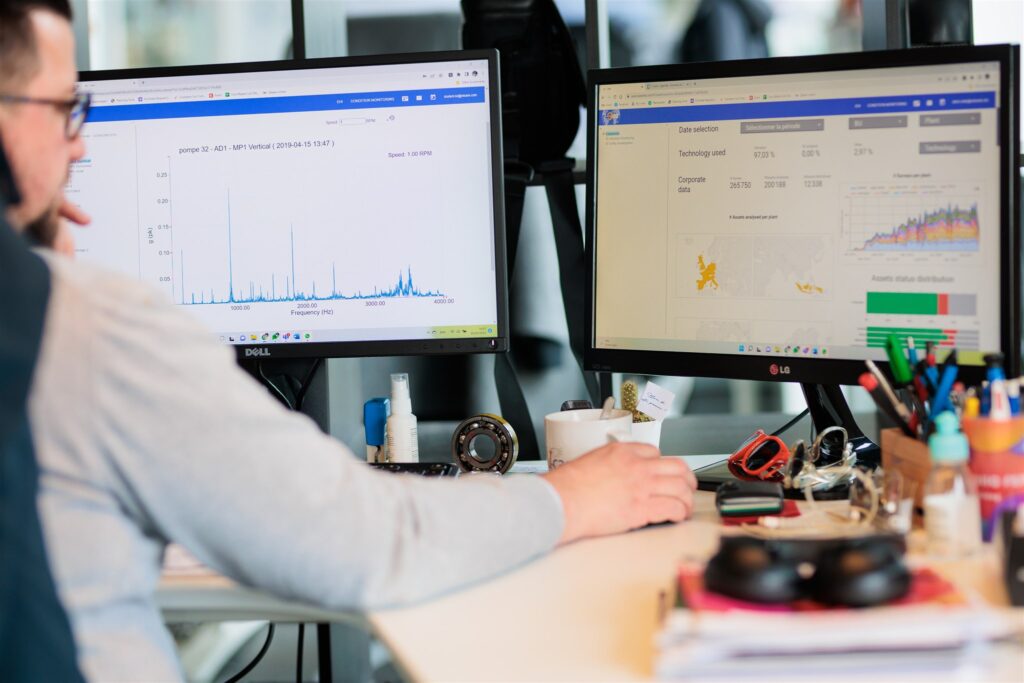
Insight has been the impetus for manufacturing evolution, and yet the vast majority of operational data is still not leveraged to perform and interpret analytics. This inaction is causing industrial firms to miss a huge opportunity to expand insight and optimize operations. Industrial control data runs the gamut from vibration readings to statistics on granular activities, e.g. how much time a particular piece of equipment spends cutting (in metal-to-metal contact).
A sensor might monitor and report on vibration limits for a certain machine or one of its parts. PLC data from that machine might provide notification that a fault is occurring in production. When data from industrial equipment is collected, aggregated, and most importantly, analysed and interpreted, manufacturers are empowered to convert that data into information and turn information into insight.
Yet, many industrial leaders currently don’t understand the value of multiple point-source aggregation and analysis for informing tasks ranging from predicting failures and resolving operating bottlenecks to tracking and optimizing daily production schedules. Some don’t even realize how much information can be captured. In the least- efficient scenario, personnel is still managing equipment, and its maintenance and operation, using hand-written checklists.
IMPEDIMENTS TO OVERCOME
Given all these benefits of data and its usage, why are facility personnel, from plant leaders to shop floor managers, still questioning its value?
Engineers and other operating staff and management don’t have time to gather data, nor do they understand the value of analytics to interpret the data. Exacerbating the situation, many view machinery as the core value in any facility. They approach information technology with distrust.
On the other side of the facility, internal teams and/or managers may already be using analytics tools for varied reasons, whether preparing sales forecasts from historical data or benchmarking product output numbers against expectations. They may recognize that each piece of their firm’s equipment produces data, but their expertise is in organizing and interpreting data for business purposes, not in gathering it from machinery.
No one, from the boardroom to the plant floor, is sure what to do with the information they could extract from sensors and PLCs, so no one acts. This impasse is being played out daily, all over the world, and it is costing companies a fortune.
Working with outside experts, shrewd facility operators are now making productive use of this data, interpreting and applying it to achieve the predictability and reduced failure rates critical to operational excellence. In short, they are moving beyond just predicting problems to identifying solutions, and data analytics is leading the way.
MANUFACTURING IN 2021: ISSUES AND EXPOSURE
Modern manufacturers are under pressure from a variety of threats, finding their profits squeezed by rising materials costs, broken supply chains, resulting in materials shortages, and worker-availability crises. At the same time, they are struggling to evade new emerging threats, such as ransomware and theft of intellectual property.
Even as they attempt to address these threats (or at least mitigate the damage they cause), manufacturing leaders are facing lost production hours due to downtime (323 production hours a year on average), shrinking profit margins from the competition, and ever-evolving safety and compliance mandates that can require product design and factory line changes. Faced with this onslaught of obligations, it is evident manufacturers can no longer afford to overlook any potential source of insight that can increase their operating productivity.
The good news, as research firm McKinsey noted in a recent analysis, is that two of these pressures — productivity and profitability — can be mitigated through the effective use of manufacturing data analytics. Furthermore, while much has been written about how automation can potentially increase liability (think failing robot cars), effective data analytics are a key to reducing it.
IDENTIFYING THE NEED
In the quest for solutions to these challenges, I-care analysts have developed a list of questions that decision-makers should ask themselves.
The answers will help them identify where they fall, in terms of gathering and utilizing the data available to them through industrial sensors and then putting it to work to boost their productivity and their profits.
- Can we monitor our machines and processes in real-time?
- Can we understand problems in the context of history and trends?
- Can we use this data to solve the problems we identify before they cost us money?
- Once solved, can we monitor the situation moving forward to ensure it is resolved?
- Can we measure the ROI of solving this problem?
If the answer is “No” to any of these questions, it’s time for leadership to take a deeper dive into the data available to them. They can work backward from there to understand what gaps exist in their ability to understand manufacturing data in context.
Putting historical data to productive use enables leadership to make more informed, effective decisions regarding their equipment. The outcome is predictability and reduced failure rates that are critical to operational excellence.
DATA COLLECTED BY SENSORS AND OTHER MECHANISMS: A UNIQUE OPPORTUNITY, OFTEN SQUANDERED
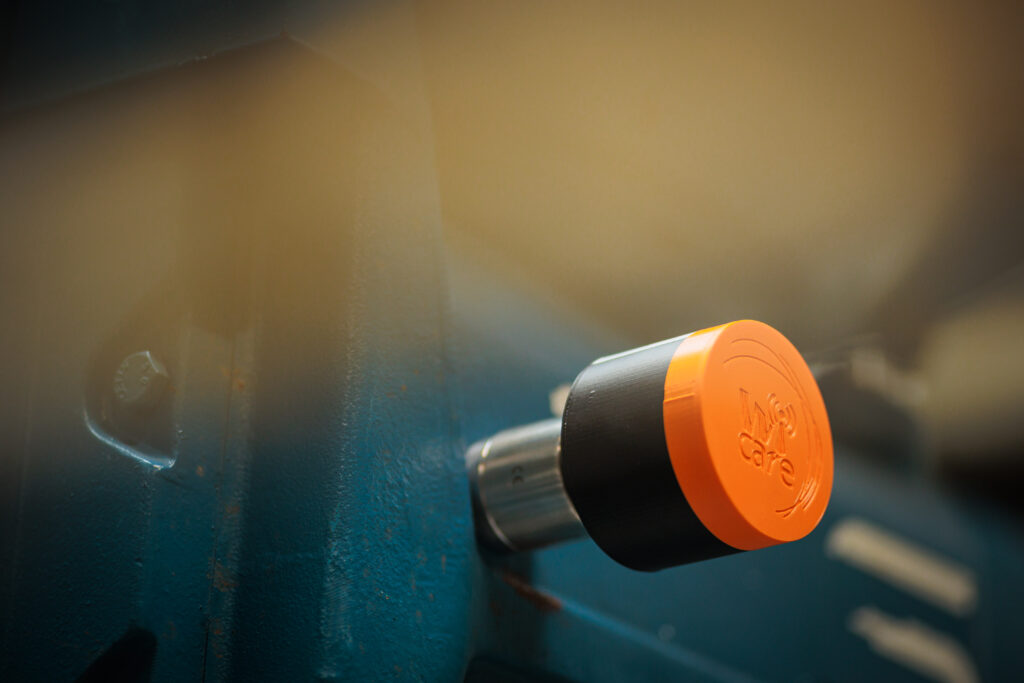
Manufacturers, and process industries, in particular, generate enormous volumes of data, but many have failed to make use of this mountain of potential intelligence. In fact, despite the broad availability of sensor data, up to 90% of the data generated in manufacturing goes unused or is even discarded. Why is this?
Historically, manufacturers have lagged behind other industries in their information technology capabilities. As a result, organizations often don’t possess the expertise to harvest, analyze and interpret data accurately. Exacerbating the issue, maintenance and plant workers, who would likely recognize the benefit they could gain from more input, don’t control whether or not the firm harvests, interprets, and applies its data. CIOs and continuous improvement personnel, despite having responsibility for ensuring optimal plant operations, are too far removed from the plant floor to recognize the benefits of data-driven analysis.
This is unfortunate because sensors can provide deeply rich insights, even translating physical actions from machines into digital signals that communicate variables such as temperature, vibration, or conductivity. Furthermore, high levels of asset ignorance paired with low levels of insight among decision-makers remain key factors in unplanned downtime.
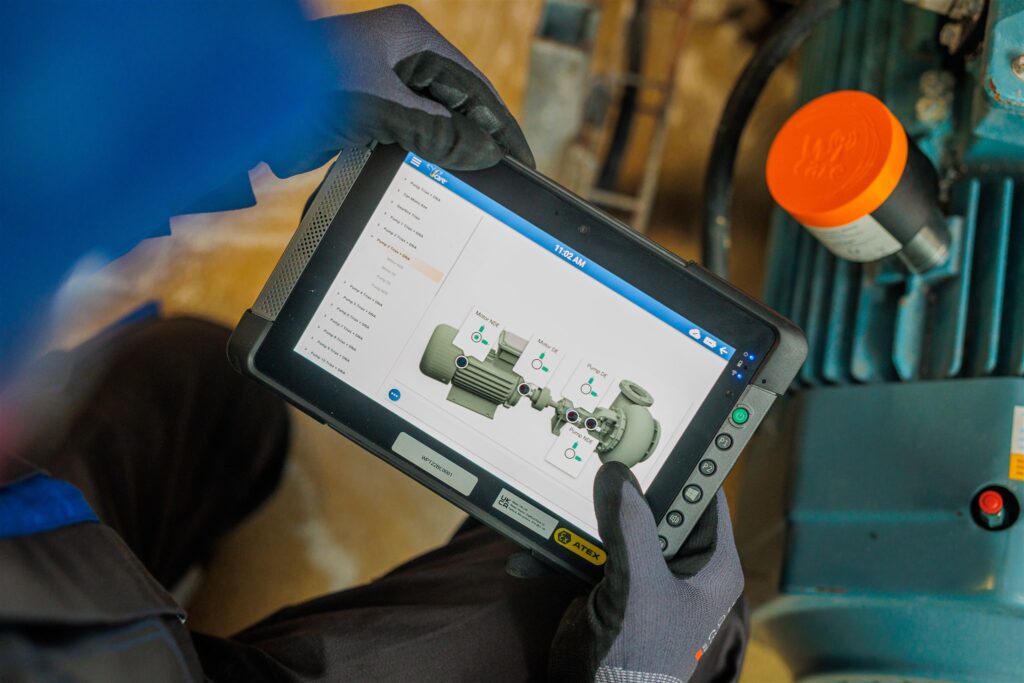
Per a study by research firm Vanson Bourne, only 31% of decision-makers have insight into when their assets are due to be upgraded; 29% know when they are scheduled for maintenance, and only 25% have total awareness of when they are due to be replaced. Perhaps even more disconcerting, a 2019 Plant Engineering Facilities Maintenance study found that 56% of operations were using a “run-to-failure” method of maintenance and therefore had no asset intelligence program or strategy. Of those who had a maintenance plan, 45% were using in-house created spreadsheets and schedules (e.g. Microsoft Excel) to manage their maintenance schedules and efforts.
MOVING FROM DATA HARVESTING TO DATA INSIGHT
These outdated approaches and attitudes are especially surprising given the value-add benefits of data collection and analysis. Advanced analytics are proven to provide meaningful insight, helping manufacturers solve previously impenetrable problems and reveal those they never knew about, such as hidden bottlenecks or unprofitable production lines. One of the key impediments our experts have identified is lack of a clear idea what data needs to be measured, collected and analyzed — and why that particular data is important (what benefit it provides).
This lack of awareness can cause a direct negative impact on the firm’s bottom line, as proactive monitoring of asset health allows operations to sustain current production goals while accomplishing performance enhancements necessary to meet evolving production targets and business commitments.
Furthermore, thanks to less expensive computational power and rapidly advancing analytics opportunities, process manufacturers can gather information from multiple data sources, analyze it and use it to uncover new ways to optimize their processes, from the sourcing of raw materials to the sale of finished products. This is generally done in one of two ways (and sometimes both).
- Technological interpretation, such as that performed by artificial intelligence (AI)
- Human evaluation and interpretation, where experts collect, process and interpret the data, which results in a more comprehensive analysis for several reasons:
- Humans have the ability to make abstract connections with which AI struggles.
- They recognize the value (and have the capability) of linking other sources, such as a computerized maintenance management system (CMMS) or enterprise resource planning (ERP) system. Aggregating data from additional sources enables companies to maximize the benefit of the data by combining sensor data with historical maintenance records.
- They can apply the results of their analysis to outside variables that factor into the equation, gaining an even broader understanding of the issues.
At this stage, augmented outcomes become possible. Consider a scenario where AI-driven algorithms are used to automatically trigger a maintenance work order in a company’s CMMS system, check the ERP system for spares on hand, and generate a purchase request for any additional parts required for routine maintenance.
At that point, the maintenance manager or other decision maker only needs to approve the items in the workflow and dispatch the appropriate technician. Such a system can have a meaningful impact on the mission-critical goal of avoiding unplanned downtime.
This example is only one of many ways data, when harvested, analyzed and interpreted, can lead to greater insight that results in less frequent equipment failure and higher uptime levels. Simply gathering data on machinery from sensors is not a useful strategy. Data that is gathered must be transformed and then applied in a purposeful manner.
AN ANALYTICS TRANSFORMATION DELIVERS RELIABLE OUTCOMES
Predicting the failure of individual parts requires high-level solutions to sift through the mountains of data to find the “signal” of a part about to fail in the “noise” of daily operation. One example is a lubrication-related failure.
Up to 80% of all bearing failures (catastrophic, functional, and premature) are lubrication-related. Predictive Maintenance (PdM) arose in response to facility operators seeking to avert these failures. However, PdM by its nature will only predict when something might happen. Depending upon the accuracy of “average” failure thresholds, PdM equips teams with information, not insight.
Without expert analysis and interpretation of available data, operators and their teams will not have all the input they need to make effective decisions. The outcome is often a false sense of security that can lead to very detrimental results. The proactive data analysis and interpretation found with prescriptive maintenance enables firms to “fine tune” each prediction, adjusting settings accordingly. Yet, even the outcomes common with prescriptive maintenance can be improved significantly with the addition of data-driven insight.
ACHIEVING THE PINNACLE OF AWARENESS
Unfortunately, the buzz surrounding AI and its ability to inform predictive and prescriptive maintenance routines has caused the manufacturing industry to move heavily in the direction of full automation. This sentiment was summarized effectively by Dan Miklovic, a principal analyst at LNS Research. In 2016, Miklovic wrote, “No longer will you need an ensemble of experts to tell you how and when to maintain your assets, as the assets themselves will tell you what they need if they are unable to fix themselves.”
Unfortunately, the buzz surrounding AI and its ability to inform predictive and prescriptive maintenance routines has caused the manufacturing industry to move heavily in the direction of full automation. This sentiment was summarized effectively by Dan Miklovic,10 a principal analyst at LNS Research. In 2016, Miklovic wrote, “No longer will you need an ensemble of experts to tell you how and when to maintain your assets, as the assets themselves will tell you what they need if they are unable to fix themselves.”
The insight derived from data collection, analysis and interpretation confers many other benefits, as well. One that is mission-critical is safety due to fewer accidents. A 2016 study designed to determine how failures of commonly used industrial equipment, such as machines, tools and material handling equipment, contributed to the chain of causality of industrial accidents and incidents.
The researchers found that 33% of industrial accidents were related to equipment failure; some with direct human consequences. In the abstract for the study, authors Dominic Bourassa, François Gauthier, and Georges Abdul-Nour noted, “Based on a case study which aimed at the analysis of an existing pulp and paper company’s accident database… results show that equipment failures had a major effect on the number and severity of accidents accounted for in the database.”
Given that production and site safety are also good for business and stock prices, safety provides a ripple effect. Other benefits are myriad, as well. From energy savings and emissions reductions resulting from better asset performance to greater uptime that minimizes the need to maintain a sizeable spare parts inventory, the benefits are tangible and quantifiable. Even supply chain management becomes more effective with data- driven asset management.
NEXT STEPS: GETTING TO YES
If you are reading this article and do not have corporate decision-making authority, you may now be wondering, “How do I get upper management on board with this idea?” Our company has deep expertise in helping companies “get to yes” with data analysis and interpretation projects that provide the benefits stated above. From red tape to outdated attitudes, we are happy to assist.
Our experience includes successful engagements with other organizations that we can leverage to provide even more support for the value of data-driven insight. We can also help your firm develop a complete roll-out program, including stakeholder meetings, the development of a “quick win” project, and even a pilot program. All of these methodologies have proven benefits.
In summary, although choosing the most appropriate analytics and applying them to drive insight for better decision-making requires specialized expertise, the results can be significant. By leveraging machine learning alone, one manufacturer reduced downtime on a robotic manufacturing line by 50% — and increased performance by 25%9. Imagine how much more efficient factories would become with the additional boost of human insight, analysis and interpretation.
Furthermore, accurate insight for decision making buys one of the most valuable benefits of all — time. As failure occurs less frequently, engineers are able to make incremental improvements that further boost uptime and drive profitability.
ABOUT I-CARE
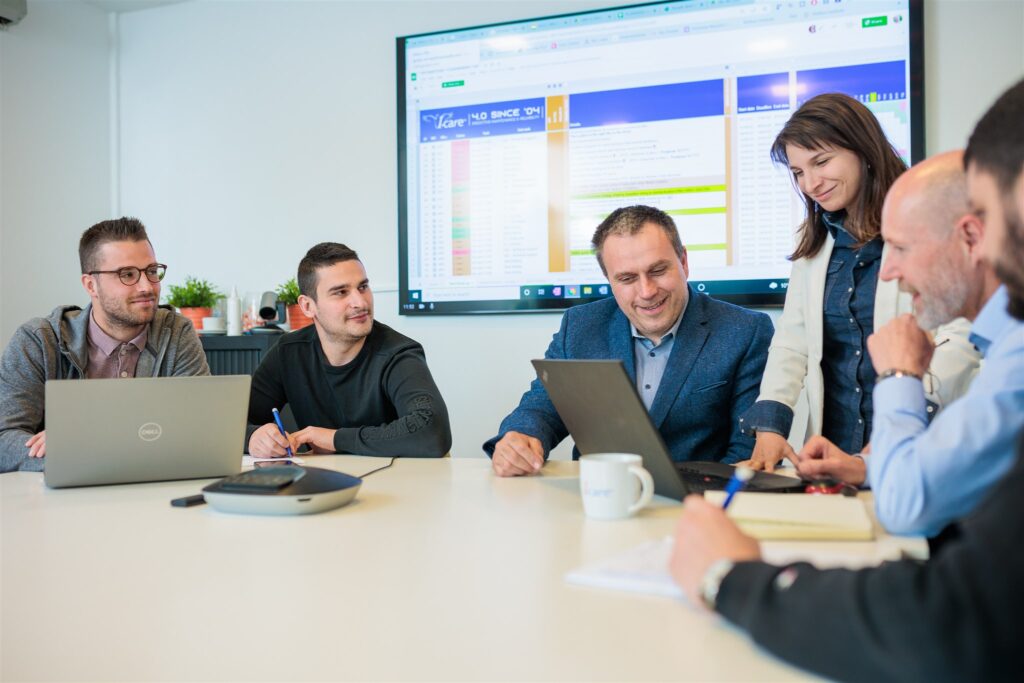
I-care™ is a global leader in predictive and prescriptive maintenance. Our AI (augmented intelligence)- powered and data-driven solutions predict industrial failures before anyone else. With our innovative methods, products and services, we take care of thousands of assets and processes for clients around the world, and we help them reduce risks for safer, more productive, and more sustainable industries.
Founded in 2004 and based in Belgium, I-care employs more than 500 people and serves customers in 26 countries (Europe, USA, and Asia- Pacific). I-care was named Business of the Year 2020 by Ernst and Young, an honor for which Belgian Prime Minister Alexander De Croo bestowed a trophy to I-care Founder and CEO Fabrice Brion.
ABOUT OUR AUTHORS

VIRGINIE DE STEXHE | CHIEF PROJECT OFFICER
She became I-care’s Chief Project and Data Officer in 2021.
Virginie de Stexhe holds a Master’s Degree in Industrial Engineering from HELHa, Mons (Belgium).
Virginie has managed significant projects, including the development of the second generation of I-see products or the development of the company’s first corporate asset model contract.
Virginie de Stexhe started her career as a sales engineer at Schneider Electric.
She joined I-care in 2008 and is the company’s 6th hire. She was the first reliability engineer to perform predictive maintenance in combination with prescriptive maintenance for a large industrial client. She oversaw the supervision and execution of several prescriptive maintenance projects for large and critical industries and became Reliability Director in 2012.
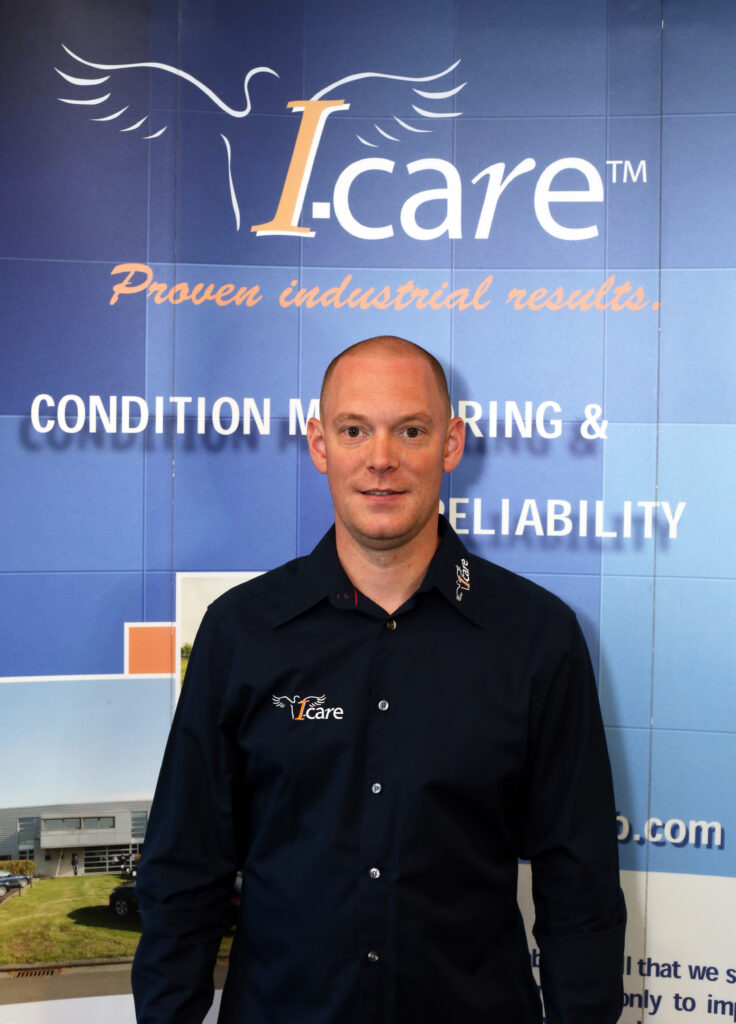
PIETER VAN CAMP | CHIEF COMMERCIAL OFFICER
Pieter Van Camp joined I-care group in 2015 as a Corporate Business Development Manager.
He was promoted to Chief Commercial Officer in 2018. Pieter has over 20 years of experience in predictive maintenance. Following his thesis on the “Implementation of a predictive maintenance approach on robots in the automotive industry”, he started his career as a predictive maintenance expert with Emerson, coaching industrial and commercial customers to improve their reliability and maintenance processes, using digital technologies.
Pieter Van Camp holds a Master of Science in Electromechanical Engineering from Internationale Hogeschool Leuven (Belgium).